Embracing transformation: how big data, AI and digitization are changing cell and gene therapy manufacture
Cell & Gene Therapy Insights 2021; 7(4), 503–518
10.18609/cgti.2021.074
US: My name is Umay Saplakoglu, and I am the Chief Digital Officer for Cytiva. We have a powerful topic today, but a little bit of an under-utilized one for cell and gene therapies: digital technologies.
Experts say we are going through the Fourth Industrial Revolution, and it is hitting every industry a little bit differently. A lot of manufacturing solutions are becoming fully automated. Data management, data access, and data analytics are bringing us to a predictive and adaptive manufacturing environment, and all of this is falls under the phrase “digital transformation”.
We have four experts here today from academia and industry to help us understand what digital transformation means for cell and gene therapy, how is it being leveraged, and what that means for the future. Let’s begin with our first question.
Q What is digital transformation, what does it mean to you, and what type of problems is it solving for cell and gene therapies?
TK: First and foremost, it is important that we recognize that data is an asset. It becomes useful when it can be shared across an organization, and be applied from every aspect – research and discovery through to process analysis and automation, and into supply chain.
A key factor is transforming the data to be digital, and to be able to be shared across the enterprise and converted into useful knowledge that solves relevant business problems.
DM: For me it is really about smart biomanufacturing. The Catapult is a technology innovation center, so we are always looking to see how new innovations can be brought in to improve product manufacture and process development.
What we are looking at is how you can use technologies like advanced sensors, and how you can look at information technology infrastructure such as cloud computing, in order to generate, collect and interpret relatively large amounts of product and process related data. Then, to try and use this to build an environment where you can control and manage that data in a highly efficient way, and get highly representative and reproducible processes which can control a lot of the variability we see when we are trying to make these therapies.
KR: From my point of view in CMaT, which is the Cell Manufacturing Technology Center, and beyond, digital transformation really means impact, cost, access, quality, and productivity. In many ways this is not just process and product data, or analytical data, but also data from the supply chain, data from the whole instrumentation, and data from workforce training. All of this needs to be integrated to create a combined solution for the whole value chain.
That in turn increases production capacity and on-time delivery, reduces batch failure, increases quality, and ultimately reduces cost and improves access. That is what digital transformation is to me.
SS: I agree that the data-driven component of this is really important, because once you have that data what you can do with it is pretty much endless. In our situation it actually starts with something a little simpler than that, which is going paperless.
In our lab right now, a lot of our work is still driven by paper. Trying to get the folks in the lab to make that transition to a digital platform, to not writing things down, and to trusting the technology will work for them, is really a big shift. For digital transformation then, it is really thinking about how they just take a step out of what their normal day-to-day is, and move into that next phase.
Q How early do you think we should start moving towards digital transformation? What are the costs that would be involved, and what about return on investment (ROI)? What is the first step?
SS: From a cost perspective, something that has been useful to us is thinking about this from a perspective of using services. Not necessarily buying a big package or tool that you have to figure out how to get installed and get running, but rather just using a tool that you can pay for as you are using it; especially the cloud-based options. That has been really helpful to reduce that cost and overcome one of those barriers to getting involved.
When considering ROI, one of the things we are looking at is those small things that add up. Often ROI is looked at as this big picture item you can quantify across a huge set of activities. We are looking at small things like the hours that are spent creating reports, the hours that are spent going to a binder and finding the right information around the lab, and looking at how much more we will be able to improve efficiency and improve on workflow just by making those changes.
As far as some of those steps to get involved, I think one of the first, most important things is setting out what you want to accomplish. Then getting buy-in into from the people who are doing the work – not just someone who is envisioning some great technology, but rather the folks who are doing this day to day, so they can understand and get involved in making this shift.
KR: When do you start? As early as you can.
It should not be just an industry thing; it needs to be in the labs of individual investigators at every university. You start by creating that mindset in the trainees and the graduate students – that we are in a very different world, and this allows us to increase reproducibility. We will be able to mine back and identify problems much more easily than we ever could, without going through piles and piles of lab notebooks without any search facility.
The cost at that point is fairly minimal. If you build that culture up, that minimal cost then propagates through it, instead of trying to transform a whole system at a time, which is much more expensive. If we can build that culture of digitalizing things and looking at data, data mining, and data management, much earlier in the value chain and in the whole timeline, then it is not going to be a lot of cost but a lot of ROI.
Cost comes into the whole system. If you reduce batch failures, increase reproducibility, and you are able to pinpoint where your problem came from much earlier and faster, that is a huge reduction in man-hours, cost, and risk. These often do not get monetized into an ROI, but they should be. These are much bigger ROIs than whether I increased my profit or not.
DM: If digitalization has benefits, then I would have to agree with Krish that you want to start on that journey as early as you possibly can. But it is not the kind of thing where a company can say “okay, next week we are going to digitize our manufacturing process”. It is quite a long journey. Individual companies and organizations have to decide what digitalization means for them.
As Scott said, digitalization could be implementing electronic systems so you are getting rid of your paper-based systems, which is an important first step in thinking about how you are going to manage all of the data you are generating. It could be setting up a full manufacturing enterprise system where you are trying to combine your warehouse management system, your LIM system, your building management system, and your quality system, all into one usable chunk.
Something that has always been very close to my heart is thinking about how you use all of this information for advanced bioprocess control, and for improving the bioprocessing, so that we can get higher quality products being manufactured. But it is a journey, and we need steps.
Even if you are looking at things like advanced process control, your first step is to try and build more of a mechanistic understanding of your product. You need to define what it is you want to be able to measure within that process. That is then going to inform what kind of sensor technology or analytics you can apply to make those measurements. You have got to think about how you are going to integrate these analytics or sensor technologies into your process, because a lot of the processes we have are not designed to integrate with other technologies. We have a bit of a step change there in terms of technology integration.
And we have got to think how we are going to manage the data. We are lucky to have Theresa on this roundtable, because the potentially huge amount of data we are spitting out has to be managed, processed, and presented in a way that you can ultimately go on to use.
Then, you have to think about the physical systems you are going to use. Even with one element, like advanced process control, it is not a simple process. You have to think about the steps you want to take. Think about how that can be done in a quality by design framework, so that you can make sure you are starting out with the end goal in mind, and building in the steps to make sure it is achievable, because ultimately it is about creating better, higher quality products.
Q Are there any particular elements of the digital ecosystem that are currently overlooked or undervalued by the cell and gene therapy field?
DM: The thing I think is undervalued is data management. We produce data in a lot of different ways, and we don’t often manage that data to the best of our abilities. We could be performing univariant analysis where we are just looking at cause and effect; at what is going on within a system. We have siloed data, and we are thinking about how we apply that to multivariant data analysis, or how this could be used for computational modelling. It is often just sitting there on a system, and used in not necessarily the most meaningful way.
There are a lot of bugbears around data that I have. You have issues like unique file formats, which make taking data and using it in a really meaningful way difficult. I am also shocked that we have still got equipment that you can’t network. You have to transfer data with a USB, which means someone has to physically go and take that data to a computer, and start to copy and paste. Copying and pasting is prone to errors. We need better systems for aggregating data and allowing us to get management over all of our data if we are going to make real, meaningful use of it.
KR: In my opinion there are quite a few areas that have been hugely undervalued. I totally agree with Damian that data management is a huge area.
We have not been thinking about cell and gene therapy manufacturing as a system. There are many components to gathering data. One of the key areas that the industry is gradually waking up to is supply chain management and logistics. There is huge amount of data there we have never really thought of, or looked at.
Another area that is very early on is doing a lot of measurements on your processes and your products, and longitudinal measurements on process and products. This has been largely ignored by the field. Everyone is rushing in and asking when they can get their first-in-human clinical trial, get an IPO out, and get that first product on the market, without understanding the product or the process. Then, it is irreproducible if you want to make it in multiple sites.
Gathering as much data as you can from the process product side is key. Also, when you get to clinical trial, very little data is actually captured from clinical trials these days. Gather as much data as you can, then as Damian said, think about putting it into that big data management framework, and ask what you can do with this data. Additionally, bringing in this peripheral data of supply chain and logistics would really enrich the field.
SS: As Damian said, a lot of those old processes, whether it is the file formats or connectivity of the instruments, seem to be difficult. I have also seen a lot of use of in-house technologies. That is great, and it works for what it needs to work for, but supporting that long-term – as well as sharing across the community – is not realistic.
Having more tools that can be shared across the space, and thinking about things in a way where if you spend time looking at the data and coming up with a way to assess something or report on something you can just share that capability across the community, would help tremendously. I think everyone is probably reinventing the wheel.
When it comes to engaging with the quality and regulatory side of things, that is another opportunity for bringing those folks into the technology space up front as opposed to on the back end. Saying “we built this technology, here is what we are doing, come assess our quality and make sure it fits into the space”, and maybe involving those regulators up front in designing those capabilities.
Q It is interesting that everybody goes to data as undervalued today – there is definitely a lot of value that is sitting within it. But what about talent? What are the specific considerations and needs around making sure workforces are sufficiently trained?
TK: There needs to be recognition that there is often a common workflow with the data analysis – starting with the original datasets, verifying the statistical accuracy of the data, being able to put the context around the metadata and information, and then being able to do appropriate model analysis of that. An aspect that is often not thought about is who the end user is, what the fundamental problem you are trying to solve is, and how to translate that information in a way that those users can act on it.
We often talk about augmented intelligence tools. The reason I bring this up is I am thinking about platforms that allow ease of data integration, ease of being able to do the model analysis, and then being able to repurpose those outputs systematically, moving forward. If you take that as a foundation, training the talent is about enabling them to build the system. That would be on your data informatics side, then of course you have the data scientists who would be addressing the new algorithms, and so on.
An area that is really underexploited is the users, and the application and use of the data analytics. Particularly looking at the cell and gene therapy community, being able to take these outputs and realize how they can drive better decision making from the user is overlooked.
From the talent side there is real opportunity in the field to get everyone onto the same vantage point of thinking about a workflow, and what capabilities are needed in order to be able to act on that workflow, and convert it. Whether it is in the research environment, the process environment, or in the strategic and supply chain aspects of the community.
We need to think about what can be automated and what should be automated, then convert the tools in a way that is very visual – consider how we look at data and interactions of data, and how we can put it in a visual form. If I am in a process control environment, I need to be very easily able to tell whether something is or isn’t within control. There is a whole visualization aspect to consider, and thinking about the users and what problems they are engaged with.
Then there is a whole other level of not just thinking about solving a single relationship, because in reality we are optimizing many things simultaneously. You have multi-objective, multi-response optimization. You need people to have that toolset so that they, as a domain expert, know what they need to control, or predict, or forecast, and they can put those tools together in a way that enables them to act.
KR: One of the greatest needs in this field is considering how we educate our work force, and the emerging trainers in the data science, data management, and data analytics space. We have recent funding from the National Science Foundation to put together a roadmap on how we can bring the workforce up to speed.
In addition, the needs and skillsets are going to be different for different levels of trainees and workers. The entry level trainees coming from two-year colleges, technical colleges, or high schools, need to know a certain set of skills. Then there are the users and the developers, as Theresa mentioned.
It has to be very multidisciplinary training. It is not just training in a whole bunch of algorithms, and how to manage big data and run the algorithms. Folks need to understand the biology behind it, and the process behind it. What is the product, and what does the data even mean? You need to have the informatics aspect, the cell biology aspect, and the physiology aspect. You cannot train this group of future workers and scientists on the data management alone.
On the other hand, the clinicians, cell biologists, and chemical engineers need to learn how to use that data, how to integrate data, and – at least at a basic level – to understand what this analysis is doing. Most of the time we will click on a software and out comes our data. “Here is my data. Why is it that way? Because the software told me so”. That is no longer an acceptable answer.
We need to be able to have these folks talk very different languages at the same time, and understand what is behind that data analysis, without overwhelming them. If it becomes too complicated, then you will have an aversion to taking that technology in.
SS: That is a great point. With the technologists and the folks who are at the bench, having a way they can talk to each other is really important. It is one of the things we have seen in cases in the past, where you have some sort of IT support for a system, but not necessarily IT support for the users.
Having that partnership lets the folks on the ground doing the work reach out and say I am getting ready to do this thing with the technology, is this the best way to do it? It really helps enable them to make better decisions, and to do more innovative things with the technology itself. At the same time, it helps make sure that you are not going down the path of bad habits and not doing the right thing. The more we can design the technology to make it hard to do the wrong thing, the better it is going to be.
Whether it is a technology partner, or more of a transformation or change management partner, there is some role that has to be there to help make folks who are doing the day to day more comfortable with that shift. Part of it is the tool, and part of it is making sure there is a person connected to that tool, who is not only just supporting that tool in the back end, but who can interface with the person using it.
Q How do we implement? The full and efficient realization of digital transformation in the field will clearly require a highly collaborative approach. What are some of the current examples of such partnerships, and where specifically should further collaboration efforts be focused?
TK: Krish could respond to this, as the National Science Foundation’s Engineering Research Center (NSF ERC) for cell manufacturing technologies is an outstanding example of bringing together domain experts across the entire value chain that can engage, and includes those who have the biologics expertise, those who have the clinical expertise, and those that have the data analytics expertise but may not have the biologics expertise. Being able to have a common language and identify the problems that are worth solving, then engaging with those collaborative programs to move forward, is crucial. CMaT is an excellent example of that collaboration.
KR: Within the context of CMaT, which is a nine university, three international center consortium, we have been able to work with not just academic folks but also government folks, standards development agencies, regulatory agencies, and industry, to really ask these questions about where the pain points are, and where we need to provide solutions. This is both from a workforce training standpoint, as well as a research standpoint.
One example is our partnership with Theresa, who works to understand the process and product data. We also have partnerships with Cytiva in creating a center-wide batch recording system. Doing that across nine universities is not a trivial task.
Then, partnering with AWS, we take a lot of the data being generated all over the country and internationally, and bring it into one single cloud platform where anyone from any of these nine institutions, or our international partners, can access , compute and share that data.
These are some of the partnerships we are creating, where we are understanding what the need is first, and then working with our industry and academic partners to bring that solution together. Creating that network of experts allows us to build the solution from the ground up, and customize the solution to our needs.
This cross talk is very critical. Not just for us as users, but also for the folks who are developing the solutions. Once you hit the ground, there are a lot of nuances to the solutions, and it is not a one size fits all situation. Customizability is going to be critical for those who succeed and those who fail in providing these digital solutions.
SS: There is definitely power in numbers, whether that is working with a partner like Cytiva, or any other person who will be in this space. The more people we have working together on sharing the challenges we have and the ways we can solve those challenges, the better. As Krish said, you can’t always solve that problem in the same way for every place. But if we can work together as a community to address what those concerns are and try to come up with some type of similar solution, I think that helps tremendously to move us forward.
Q US: Let’s look more closely at a few specific aspects of this, beginning from the transition from paper to digital batch records. Just how big an undertaking is this, and what are the main obstacles for digital transformation in this area?
SS: It is a big change, and I think change is hard in general, especially when you are working with a set of folks who might not be very familiar with the technology. In our situation, a lot of the folks at the bench aren’t folks who are extremely familiar with it, and they have a little bit of concern. They know how to do their paper process, and they know how to do the calculations. Doing that in a digital format can be a little overwhelming.
One of the things we have found in that process is that people like to touch something. In our efforts to get from a paper approach to a digital approach, one of questions we asked is whether we want to get rid of paper altogether. We decided no, what we would try to do is minimize it, and give the folks who really want to have something in their hand when walking around from space to space a piece of paper, so they still have that with them. That can be a summary, a report that gets run, or some type of consolidation of information.
As we work through changing some of the processes from being paper-based to digital, we are not shifting the workflow tremendously, at least initially. We are trying to keep it as similar to what they are familiar with as possible, so they can focus on having the same process but shifting it from paper to digital. Work on improving the workflows will then happen later on.
That is the approach we have taken, and some of those barriers were having the right people connect. As we discussed about in the previous question, having the right people partnering with the folks who are in the lab doing the work makes a huge difference.
TK: Scott is absolutely correct – people have to recognize that this is a cultural change. It can even be a mindset of “wait, this is my data!”. People get very protective of their data, and the possibility it will be misused, or that they will somehow lose their ability to influence and control it.
You have to help people see that the power is in sharing their data, and by having it digitized they can have greater influence and impact. When they start to see that become a reality, then they are much more on board. They realize this is opening up their ability to create value in different parts of the organization where they hadn’t previously.
This cultural change aspect is paramount to have front and center. It is good to say “this is where we are going”, but you have to make it very real to the people that are on the ground and being impacted by the change. If they don’t see the benefits, it is going to take longer. If they see the benefits and you provide the ease of enabling that, that is incredibly powerful.
Choosing a couple of low hanging fruits, and having some successful pilot projects where people can see that benefit, will enable the entire organization to move forward faster.
KR: It is a big change just within our center. When we try and implement this across nine universities and 40 different laboratories at the same time, there are various amount of heartburns, and various amounts of enthusiasm. That comes from the mindset of “this is the way I have always done it “, and it is going to change some energy barrier to cross over to the other side.
But once they see the benefit, once they see that within that broader ecosystem this is where the culture is shifting, I think people are much more receptive to taking these transformations on, and really making sure that they are at par with the advancements in the field. Because this is an advancement in the field, and everyone wants to be there at the cutting edge. Education takes a little time, but providing them with that value proposition is really critical.
Q How can in-time analytics, enabling real-time feedback loops and IPC impact your cell and gene therapy production workflow and process in practice?
DM: One of the first points is that we have got variability in our manufacturing processes. We have variability in the cells, and even if you are doing viral vector manufacturing with a cell line, you have still got variability in that mammalian cell line. We have variability coming in from raw materials, cytokines, growth factors, monoclonal antibodies, and specialist consumables we are using in the process, all of which can have batch-to-batch variability.
We are putting all of this variability in, then we have relatively rigid manufacturing processes, and then we are getting variability in the product coming out. The ability to monitor what is going on, preferably in real-time, using in-line sensors and in-line analytics, could be a real step forward. It is going to give us the ability to monitor those processes, and hopefully put mechanisms in place to get more control, and drive quality, into the system. However, we don’t have that many technologies available to us that are designed for cell and gene therapy bioprocessing yet.
If you are manufacturing viral vectors in a stirred tank reactor (STR), you are probably in quite a good place, because a lot of the technologies and processes are borrowed from biopharmaceutical manufacturing. Because they are digitizing more and more in the biopharmaceutical sector, and it is a big enough industrial sector, there are technologies available that you can pick up, and with a bit of tweaking, you can make them work for your system.
If you are not doing viral vector manufacturing in an STR, then you are probably in a much darker place, because the systems and technologies are likely not available to allow you to get this level of in-line monitoring and control.
To give an example, if you are doing CAR T manufacturing, there are increasing numbers of fully automated closed manufacturing systems that have been developed, and there is a lot of work in this field to develop more systems. But they are black boxes – they are designed to do a series of unit operations over and over again in exactly the same way. If you want to monitor what is going on within that system, you are really limited in your ability to do that.
This isn’t the fault of technology developers. It is a lack of talking and understanding within the field. Therapy developers need to be defining what critical process parameters they need to monitor, and the levels they need to monitor them to, so the technology developers can think about how to build sensors to allow them to do that. Then bioreactor companies can come along and see that this is going to be a big advantage because they will have a smarter bioreactor, and they can look at how they can integrate these technologies into their systems. But it needs that openness and dialogue to get started within the field to drive it, and that is really difficult.
We have a project that is just starting at the Cell and Gene Therapy Catapult where we are looking at technology integration, and we have got about 24 companies all coming together in a consortium. We can do this because we are not a therapy developer, and we have a platform process that we are willing to share data on. But putting that in place, from a contractual perspective and getting all the collaboration agreements in place, has taken nearly two years. It is incredibly slow.
If we want to see real innovation and change and get the advantages of in-process monitoring and control, we have got to decide what is pre-competitive within our field, and how we then bring companies together to start to share data and information.
KR: This is really the next frontier; this is where cell and gene therapy has to go. There are no ifs or buts about it. We are automating fixed processes, and if you have a highly variable input and a fixed process, your output has to be highly variable. That is just fundamental science.
Right now, we are generating thousands of doses that are all different from each other. That is the reality. If we want to have consistency in the critical quality attributes of the products that we are making, we have to have a dynamic process where we sense, decide, and control.
That should start at the very beginning of the process development and R&D phase. We need to be measuring enough things to really understand the process parameters and their effects, and to really understand the critical quality attributes you are getting at for your products, and what the right range of that quality attribute is.
Then, you can do real-time or pseudo real-time sensing at various intervals to understand if your process is going the right way or not. You need the ability to make decisions through the data analytics, and control things like the feed rate, the stirring rate, the IL-2 concentration, or whatever it is, to keep the product at that very specific range. One of the big benefits is often in cGMP, our processes are a week to six weeks long, depending on the cells and the indications. If I know that I am going to get a batch failure on day two, I just saved two million dollars of production run, even if I can just can the batch. I have avoided going through four weeks of the run, and then doing quality control just to find out that I have a failed batch.
Therefore there are a lot of benefits of real-time analytics, real-time sensors and process control that I think the field is gradually understanding. But as Damian mentioned, we really need to be able to focus on that, collaborate on that, multiple different technologies need to be brought in, and pre-competitive technologies need to be developed so that everyone can use them.
Q This is a perfect segue to machine learning and AI, another two buzzwords. What you think the main application areas are, and what is your definition for these words?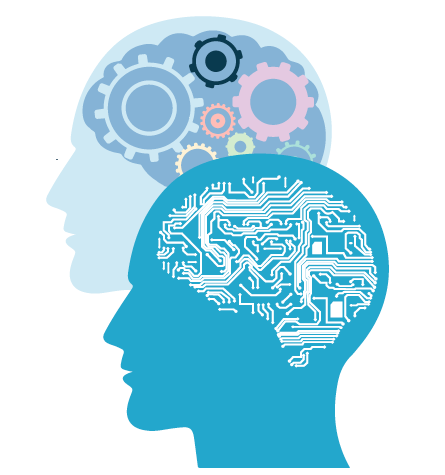
TK: In practical terms, Damian and Chris described very real programs that we are engaged in right now. I couldn’t agree more with Krish’s comment about starting in that research environment and taking the power of the elements that Damian is considering – raw material variability and cell variability – and the potential controls within the manufacturing process. Being able to look at all of those inputs in the context of the output responses that you are trying to control early in the research development is extremely useful. Having this information in a digitized form means you can then go and analyze it to understand what is not working. And at that point, what is not working is just as powerful data as what is working.
This is another aspect of digitalization that people underestimate. When you are doing data records, there is a tendency to keep a focus on a single hypothesis, and you are then looking for where that successful hit was. But when you have the digital data, you can exploit the places where you aren’t successful or where treatment isn’t effective, and that will further guide your next analysis.
Getting back to machine learning and AI, it is about taking the power of the data that you have, analyzing it, and being able to identify the driving variables or key features, and what the relationships between those features are. If I want a robust process, I typically don’t want to be controlling 100 things simultaneously. I want to know which critical set of process controls and material attributes are important.
We want to be able to do that initial model development, generate the hypothesis and understanding, then be able to exploit that and pinpoint the areas where we need to go and collect more information. They are precious data, and they are expensive, but it is better to do it then, than when you are going into a larger scale. Then you need to be able to repurpose that data, build on it, do the next round of collection and validation, and then optimize it.
Once that suite of knowledge is in place, you can look at that as machine learning or AI. I look at it as more augmented learning – you are using your models, and a domain expert can then ask what is this telling me, what do I hypothesize, how do I go validate, how can I further optimize, and what are the regions of the design space that we know we want to avoid?
To Krish’s point, with the learnings that come from that you may identify your optimum much earlier. Then from an efficiency of productivity standpoint, you can do things much faster. You can identify areas where you would want to stop production because you are now out of control, and the like.
Getting away from the buzzwords, the application of utilizing these predictive capabilities and optimization capability is what is key. Ask from a business standpoint what it is that you want to be able to more effectively predict and control, and what aspects impact it, so that you can go in to the manufacturing process exactly to this point, and select the right sensors. Being able to do the sensor side control in an automated way with confidence is foundational and key.
Even from a variability aspect, being able to bring in information about that data that you may not even anticipate as a source of variability could provide important answers. It could be that the conditions of the environment of the room or the cell, which people may not think are important, are in reality driving factors.
Q Finally, given your investments in digital transformation, what do you think your lab space or workplace will look like five years from today?
SS: Firstly, more streamlined. The more we can leverage technology, the more we will be able to take things that might be manual or things that don’t necessarily add a whole lot of value but need to be done, and automate them. This is about improving efficiency, and finding a way to enhance productivity and modify the workflow to be as optimal as possible.
Another thing which we talked about earlier was having more of the equipment and instrumentation connected into the workflow, so that we are getting data from those instruments. So for example, instead of writing down temperatures, temperatures are already being sent across. We are not having to stick in a USB drive, instead the data comes through automatically. Then we can begin leveraging that data up front and finding problems before they actually become a problem. That is a big part of what will continue to happen.
Any of those places where the technician in the lab can add value in those more complex situations, we should aim to have them focus on those, versus the more simple tasks that might feel like a big part of their job right now. That shift where they see that their role can be more valuable in those places where a human has to interact, and that is where their role will move to be, will be another change that will happen within five years. Any places where we can find those little steps will be helpful along the way.
I don’t think this is something that is just going to flip, and next thing you know we are going to be doing all of these things differently. Looking at a five-year period, I expect little things will happen each month, and each year, and that will get us to the point where things will look totally different. We want it to happen in this organic way.
KR: I would love to see a very seamless integration of batch records, metadata, analytical data, and also the big data –the ‘omics type of data. Plus, the seamless ability to analyze them no matter where you are in the organization.
Also, tools to better visualize things. That is still lacking, and that training is still lacking as to how to present it. Often we are overwhelmed with the data right now, and being able to extract features much more easily and be able to communicate that to others in a much easier and simpler manner is critical for the next five or ten years.
DM: We are in a fortunate position at the Cell and Gene Therapy Catapult, because we are in the process of setting up a manufacturing innovation center in the UK. What we are trying to do with this facility is take a lot of the things we have been talking about today and think about how we put those into practice.
We are going to look at having electronic data management systems, integrated for all parts of the manufacturing process within that facility. We are also going to be looking at how we implement process analytical technologies for in-process monitoring and control as part of the outputs from that facility.
If I was to look five years into the future, I would like to think we would have taken some pretty big steps, not just as a company but as a field, towards smart, controllable biomanufacturing. I would also hope that it is starting to become more widely adopted by companies in the field. The academic institutes are very good at driving innovation and we have got technology innovation centers like ours in most of the big developed countries around the world, that are very good at problem solving and thinking about how we get innovations into our manufacturing processes.
However, it needs that uptake from industry, and it needs them to be able to see the benefits of it and realize this is the path they want to go on. If we can start to get to that place in the next five years or so, I think we will be in a pretty good place going forward.
TK: To the earlier points, being able to have application expert tools available, that provide the ease of doing the analysis and visualizing the results, is a continual push on our side.
The fact that the industry is moving to a digital framework will open up incredible opportunities for the entire field. It also opens up new opportunities to look at sensor and control systems, and the design of those. We can start to look at ecosystems of different levels of hierarchy of relationship, and at biological controls that I don’t even think we have exploited. We are only starting to scratch the surface with the types of data we have available.
For a company such as ours, bringing in those domain experts that also have the data expertise to be able to put in place the right tools and systems for the industry will be an area of focus for us.
Authorship & Conflict of Interest
Contributions: All named authors take responsibility for the integrity of the work as a whole, and have given their approval for this version to be published.
Acknowledgements: None.
Disclosure and potential conflicts of interest: S Sobecki declares that VUMC has a partnership with Cytiva (formerly GEHC) involving one of the projects related to work in the Stem Cell Lab. T Kotanchek discloses the following roles: CMAT Industry/Practitioner Advisory Board, an unpaid board position as Chair of NMMB, an unpaid position as Chair of the Georigia Tech Manufacturing Institute (GTMI) External Advisory Board. T Kotanchek is the owner of Evolved Analytics LLC. K Roy declares the following patents: US Patent No. 10,799,246, Awarded October 13, 2020, Methods, compositions, and devices for the occlusion of cavities and passageways; US Patent No. 10,792,044, Awarded October 6, 2020, Methods, compositions, and devices for the occlusion of cavities and passageways; US Patent No. 10,675,038, Awarded June 9, 2020, Compositions and devices for the occlusion of cavities and passageways; US Patent No.10,591,403, Awarded March 17, 2020, Multiplexed analysis of cell-material niches; US Patent No.9,180,102, Awarded November 10, 2015 , Methods for fabricating nano and microparticles for drug delivery ; US Patent No. 9,096,830, Awarded August 4, 2015, Multi-Layered Hydrogel Constructs and Associated Methods; US Patent No.8,399,025, Awarded March 19, 2013 Polyamine modified particles; US Patent No. 6,475,995, Awarded Nov 5, 2002 Oral Delivery of Nucleic Acid Vaccines by Particulate Complexes; US Patent No. 5,972,707, Awarded Oct 26, 1999 Gene Delivery System. K Roy declares the following filed patents and disclosures: US Patent Application 17/046,163 “In Vitro, Multi-Niche, Bone Marrow-on-a-Chip” Filed: October 8, 2020; US Patent App. 16/343,869, Methods and Systems for T Cell Expansion, GTRC ID 7434; PCT Application No.: PCT/US2018/025827: Synthetic Particle Antibody Compositions and Uses Thereof, GTRC ID 7554; US Patent Application 15/766,657 “Methods for Generating Functional Therapeutic B. Cells Ex Vivo” Filed: April 6, 2018, Provisional Patent Filed: Method for Reducing Toxicity of Cationic Polymeric Nanoparticles Through Imidazole Modification, US Application No.: 62/403,644, Filed: 3 October 2016; Patent Filed: 61/328,339, Method of Producing Antigen-Specific Cells, Filed, April 2010; Patent Filed: USPTO # 20100311654, Modified Polysaccharide-Based Delivery of Nucleic Acids, March 9, 2010; Patent Filed: USPTO # 2010031866, Hydrogels for Combinatorial Delivery of Immune-Modulating Bio Molecules, April 2010; Patent filed: USPTO # 20110206617, Modified polysaccharides for drug and contrast agent delivery, February 2010; Patent Filed: USPTO # 20100010102Triggered release of drugs from polymer particles, July 9, 2009; Patent Filed: USPTO 20070014752 Surface functionalization of polymeric materials, July 7, 2006; Provisional Patent Filed: 065715.0114 (OTC-5009-Roy) Systems and Methods for the Production of Differentiated Cells, April 2005; Patent Filed: USPTO # 20040147466 Nucleic acid delivery formulations. K Roy discloses participation on the following Boards: Johns Hopkins ImmunoEngineering Center, MIT-Singapore Appliance for Cell Therapy Manufacturing, University of Miami Stem Cell Center, Children’s Hospital of Atlanta Cell Therapy Center. K Roy declares that the Center for Cell Manufacturing Technologies has received the following as in-kind contributions for their membership in the NSFCenter: Cytiva, Axion Biosystems, Terumo BCT, IsoPlexis, Beckman Coulter, Rooster Bio, Etaluma, Enable Life Sciences, Evolved Analytics, Acea Biosciences (Agilent), Janssen, Lonza, MilliporeSigma, Aruna Bio, Bristol Myers Squibb, Century Therapeutics, Rubhu Biologics, Sangamo Therapeutics, Lucid Scientific, Veranome Biosystems (Applied Materials), Nucleus Biologics, XCell, Scientific Bioprocessing, Synthego.
Funding declaration: S Sobecki declares that VUMC has a partnership with Cytiva (formerly GEHC) involving one of the projects related to work in the Stem Cell Lab. The authors received no financial support for the research, authorship and/or publication of this article. T Kotanchek declares the following licenses: DataModeler Software License from Georgia Tech Marcus center and royality free DataModeler licences from Evolved Analytics as part of an in-kind contribution to CMAT. K Roy declares NSF Engineering Research Center for Cell Manufacturing Technologies (CMaT), Grant no. NSF EEC 1648035 in relation to the current manuscript. In addition, K Roy discloses NSF Engineering Research Center for Cell Manufacturing Technologies (CMaT), Grant no. NSF EEC 1648035. K Roy has also received consulting fees from Terumo BCT, Merck, Clearview Partners and LEK Consulting. K Roy has received payment from ACS Annual Meeting and Bioprocessing Summit in relation to lectures, presentations or educational events. K Roy has recevied support for attending the following meetings: Bioprocessing Summit, ISCT Annual meeting, BMES and Biophysical Society. K Roy declares stock or stock options in the following: Astrazeneca, Antares Pharma, Gilead Sciences, Lineage Cell Therapeutics and Geron.
Article & copyright information
Copyright: Published by Cell and Gene Therapy Insights under Creative Commons License Deed CC BY NC ND 4.0 which allows anyone to copy, distribute, and transmit the article provided it is properly attributed in the manner specified below. No commercial use without permission.
Attribution: Copyright © 2019 Name INTL. Published by Cell and Gene Therapy Insights under Creative Commons License Deed CC BY NC ND 4.0.
Article source: Copyright © 2021 Cytiva. Published by Cell and Gene Therapy Insights under Creative Commons License Deed CC BY NC
ND 4.0.
Article source: This is a transcript of an Expert Roundtable, which can be found here. Publication date: 14 May 2021.